AI As A Force For Good
Horasis held its first digital Extra-Ordinary Meeting on 1st October 2020 with the theme of Unite. Inspire. Create. The meeting brought together heads of state, prime ministers, and other government representatives and CEOs and entrepreneurs from around the world. Nine hundred speakers contributed to discuss themes spanning politics, business, and civil society across 150 sessions – the most extensive online meeting held by any organization so far.
The panel on Artificial Intelligence as a force for good took place at 10:15 CEST with Gurvinder Ahluwalia, CEO of Digital Twins Lab (USA), Rana Gujral, CEO of Behavioral Signals (USA), Ayla Annac, CEO of InvivoSciences (USA), Jean Lehmann, CEO of Cyber Capital HQ (UK), Julien Weissenberg, CEO of Visualsense AI (Switzerland), and Marta F. Belcher, Attorney, Ropes & Gray (USA).
For all its hype in post-COVID digital mania, Artificial Intelligence (AI) alone cannot complete diverse tasks: it has to be trained in a specific action domain. Its training is subject to human bias. AI can be a potent amplifier of human endeavor and can inspire our future creativity. How can we ensure it is always working for us? How can we ensure it acts ethically?
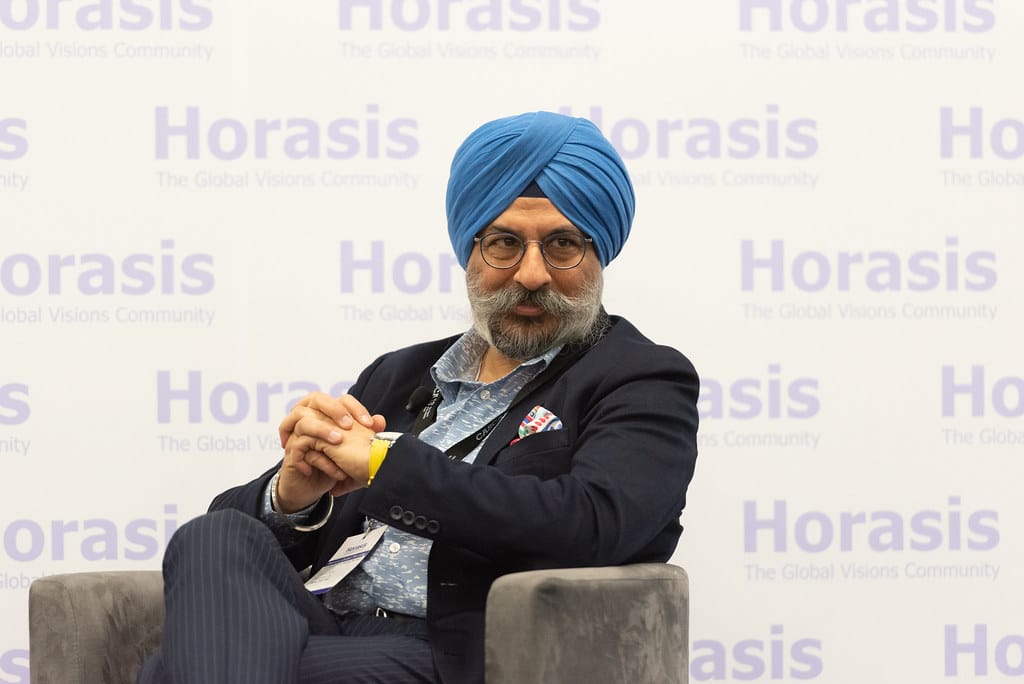
Gurvinder Ahluwalia
Gurvinder Ahluwalia, CEO of Digital Twin Labs, a US company that creates strategy and develops digital platforms and products globally based on frontier technologies including AI/ML, Blockchain, IoT, and Cloud , moderated the panel.
As AI interfaces with healthcare and medical science, laws, and ethics, capital markets, and economics, what would you characterize AI to be about? Is AI about automation, is about prediction, or something else? How should we deal with AI bias when specific algorithms that may be used in the justice system could predict that a black person is at a much higher risk of committing a crime or algorithms that may be used by employers indicating that women are underperforming relative to men? Who will govern AI to decide which patient population will get the treatment they need?
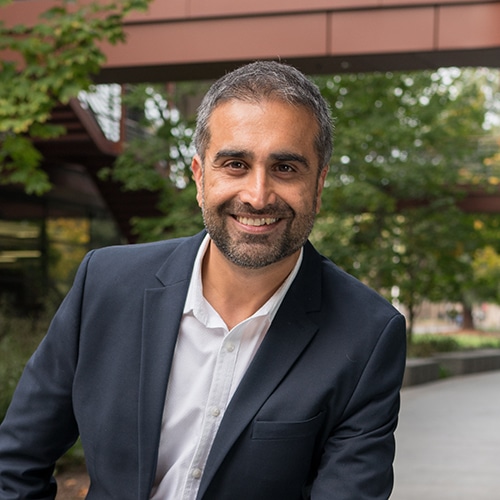
Rana Gujral
Rana Gujral highlighted what we could infer when people are having a conversation. His company, Behavioral Signals, focuses on unraveling insights from the tone of voice, such as pitch and tonal variance, tonality, among other elements. There are two main elements to consider when people have a conversation: first is understanding what the person is saying, second how the person is saying, focusing on the actual delivery of the content. Behavioral Signals focuses on the “how part” and unravels insights from the from the “how part”, such as emotional signals such as anger, happiness or sadness, behavioral signals such as engagement, empathy, politeness, to go as far as predict and unravel intent insights, for instance, a buying decision or do an action in the context of the discussion at hand. Rana’s company spent two decades developing this technology with multiple breakthroughs to maximize business conversations. Applications in financial services can help with complex conversations around the collection and various applications in human to machine context. The tone of voice can give a very accurate assessment of how a person feels, and applications are endless.
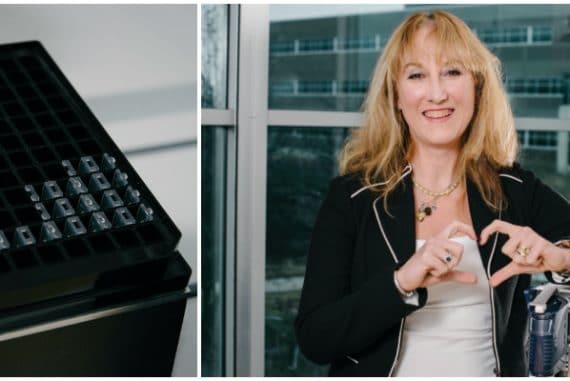
Ayla Annac
Ayla Annac’s company, InvivoSciences, focuses on novel treatments for genetic heart failure and to cure heart diseases one gene at a time. Her company can take cells directly from blood samples and create the micro human heart tissues to test drugs’ efficacy and safety, validate drug targets, repurpose and or identify new compounds. This integration with AI helps decrease drug development costs, increases the technology’s predictive power on the drug, and the matching to the population with the most efficacious precision medicine. And in the integration platform, we train the AI with information coming from the donor, which is the gold standard. This process is very rigorous and will help develop the future generation of drugs. You can classify the patient populations based on the genetically inherited disease. Heart failure is the number one killer around the globe. Most people are concerned, including women, children, and the older population, and it is a big issue, especially in the US. Heart failure is an even bigger concern among the cancer patients under chemotherapy and who has inherited heart failure.
Regarding developing a vaccine, AI plays a critical role in safety and efficacy and repositioning drugs much quicker and cost effectively to bring a safe trial for humans. AI best predicts the safety and effective matching to the population with limited clinical trials. AI can help to reposition a new compound as developed.
As every day continues to see cybersecurity headlines about the latest hacks, what organizations should do to build more secure and resilient systems, and how can we apply AI in Cybersecurity?
Cybersecurity is increasingly becoming on the agenda of private companies and governments. As stated by the President of Armenia, His Excellency Armen Sarkissian, during the Horasis meeting: “the world has changed in many places. If you come back in time and tell people that the global risk of being connected to the Internet would be huge, people would not believe that. This is a completely different world. Along with the nuclear threat, there is a new threat, which is the cyber threat.”
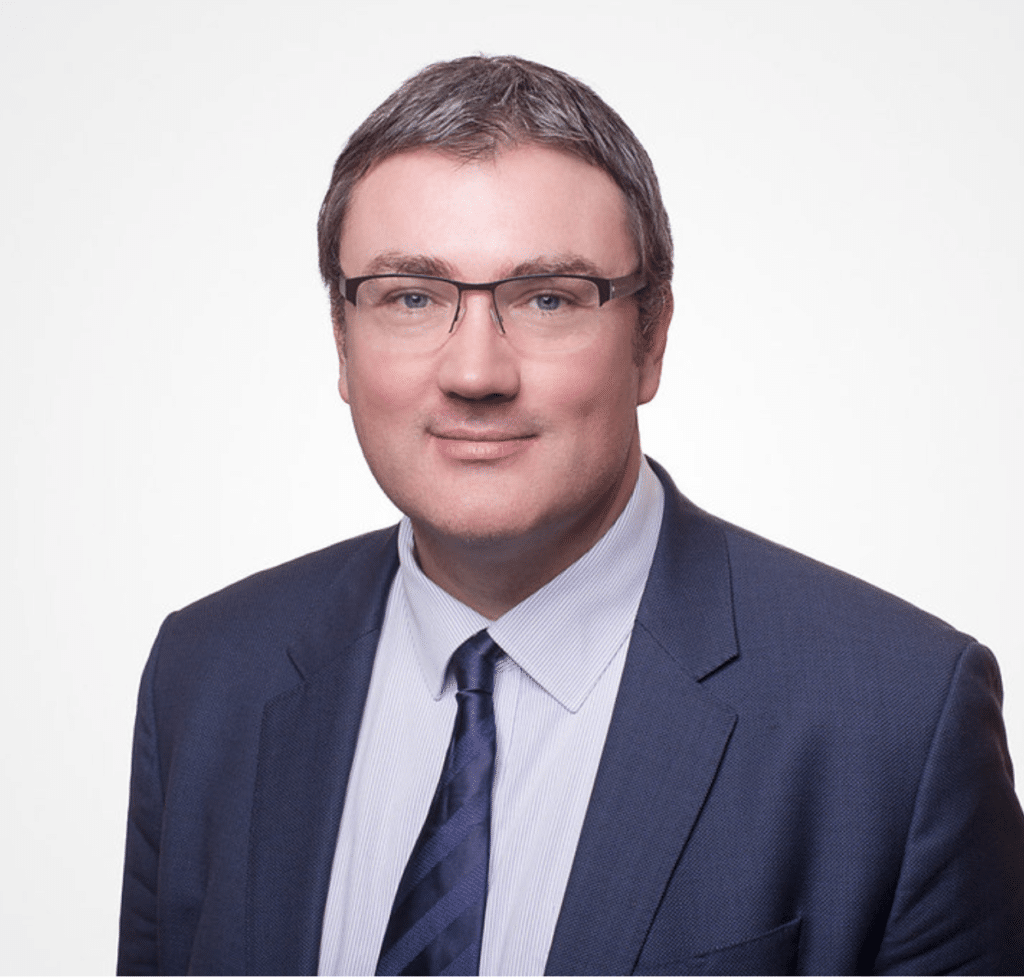
Jean Lehmann
As investments in the industry grow, cyberattacks show no signs of abating. Jean Lehmann, CEO of Cyber Capital HQ, works with the leading companies that apply artificial intelligence and machine learning to solve some of the most complex and challenging problems in Cybersecurity. Jean is generally interested in the convergence of trends and technologies, and the intersection of policy, technology, and regulation.
We observe an application of AI/ML in Cybersecurity in the prevention against zero-day threats. Traditional AV solutions maintain extensive databases of known signatures (in the form of hash) of malicious files, and new files are scanned against those databases of signatures. Zero-day threats have no known signatures. As such, they can’t be flagged as malicious by traditional/legacy AV and thus generate false negatives. Machine Learning and AI can predict and prevent zero-day threats with a very high level of accuracy. Companies like Cylance, Deep Instinct apply machine learning and deep learning to tackle this problem. Cybersecurity machine learning generations are distinguished from one another according to five primary factors, which reflect the intersection of data science and cybersecurity platforms: Runtime, Features, Datasets, Human interaction, and Goodness of Fit.
Any connected system, no matter how secure, is susceptible to cyberattacks. Threats can be both internal and external (internal threats are most common (59%), followed by external (42%) and third-parties (4%)). Cyber Capital HQ looks at implementing controls across the people, process, and technology framework to increase an organization’s resiliency. Increasing resiliency means decreasing the likelihood of a breach and the impact in case of a breach. Good Cybersecurity makes it expensive for hackers to break into a system. CCHQ aims at maximizing Return on Investment, minimize internal and external risks, increase productivity and efficiency, and save costs. Such an approach is coherent with reconciling privacy, security, and usability, building a system out of a virtuous and reinforcing feedback loop between those three notions.
Cybersecurity is a complex field, but implementing adequate cybersecurity measures and hygiene doesn’t have to be complicated. Some basic principles can protect most organizations against the majority of cyberattacks and data breaches. Had companies implemented MFA (multi-factor authentication) consistently in the last ten years, we would have seen 80% of data breaches less. Organizations that need to juggle with scale, many applications, processes, and procedures, a large user base can implement strategies, practices, policies, and technologies to gain significant efficiency and productivity. The COVID situation has also created an environment where people and companies face an ever expanding attack surface and cyber threats on one side and need to redefine ways of working on the other side. It’s never been more challenging in terms of balancing the needs for business security, employee productivity, and user experience.
Cybersecurity is a much broader issue than technology and encompasses policy, social, political, economic, societal considerations. Security should underpin and support safety. The priority should always be safety, which means that we are putting the human at the center. Making the world a safer place means putting humans at the center.
Is it true that AI can read human expressions and detect feelings and sentiments in a session like this? Is the technology close to reaching that stage?
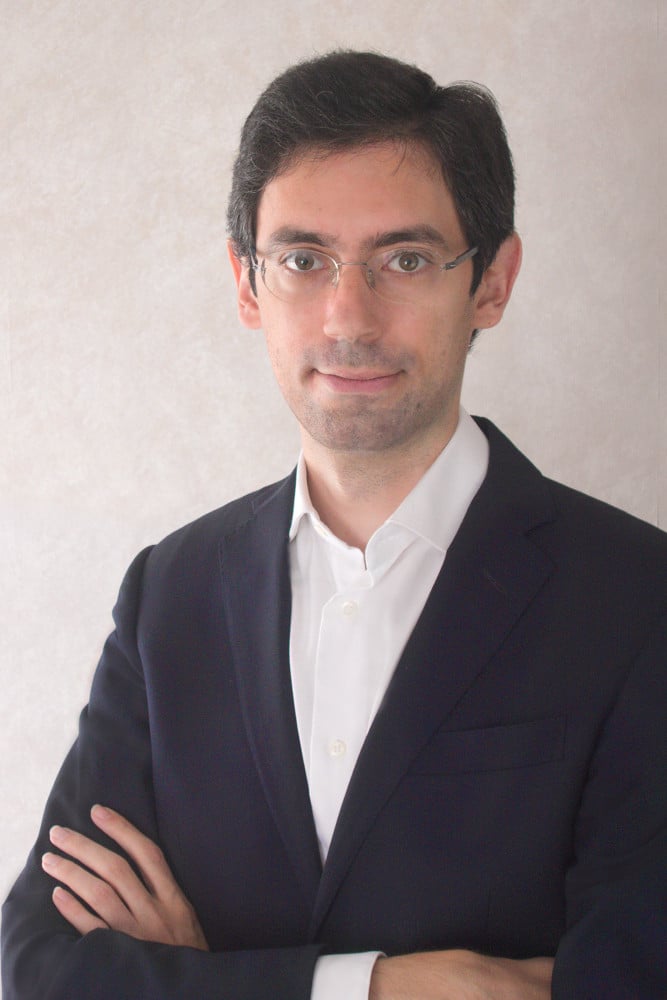
Julien Weissenberg
Julian Weissenberg, CEO of Visual Sense, is an expert focusing on computer vision and work on many different applications, like facial recognition, document analysis, infrastructure inspection, warehouse optimization, and helps companies around their data strategy algorithms to use, and due diligence on technology. The tools we have, already for quite some time, are very able to read people, not only feelings and expressions but also micromovements and amplify them. This can be used to check someone’s pulse by looking at the picture as your heartbeats. We can see the frequency of heartbeat and breathing, which may be useful to monitor a baby’s health but could also have adverse use. It’s a double edge sword as it could be used in dubious ways. The same technology can also be used by watching over by the window and trying to reconstruct the sound in the room from the vibration.
How about the legal aspects and privacy implications on total or facial recognition using AI? Could you unpack the legal elements and give us an introduction to your expertise and work?
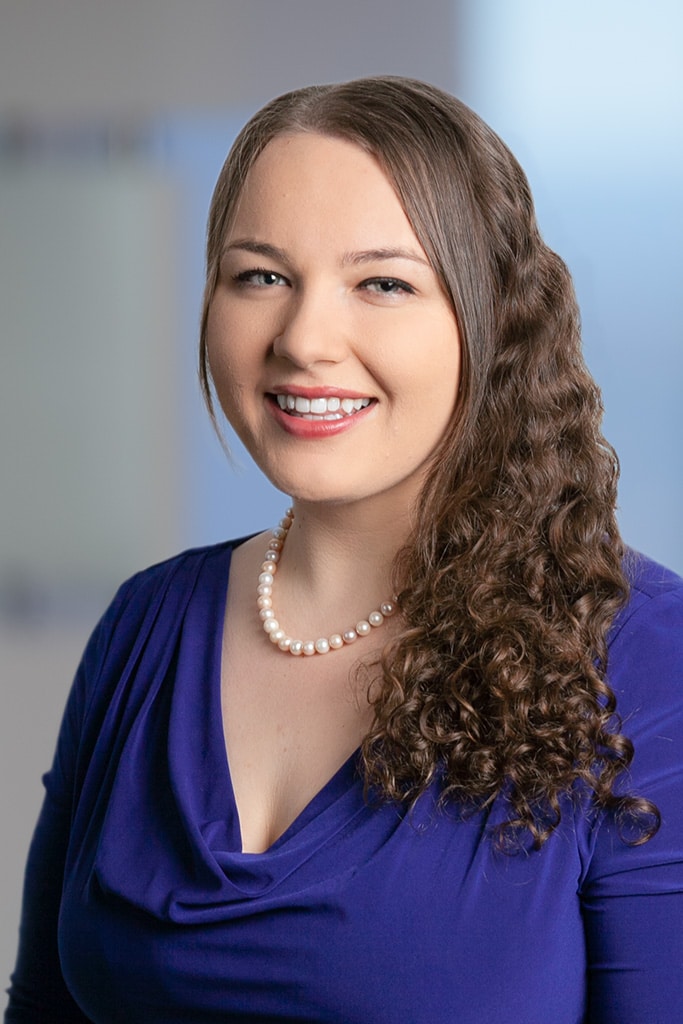
Marta Belcher
Marta Belcher is an attorney in Silicon Valley, focusing on emerging technologies. Algorithms already make so many decisions that affect daily life, for example price discrimination when you shop for airline tickets. Algorithms are also sometimes used for criminal sentencing, and the inability to get into the code to see how it is making decisions causes potential problems with transparency into how those decisions are being made.
What are the challenges of bias in AI?
In some states in the US, as part of criminal sentencing, a private party will conduct a risk assessment given to the judge to help them understand how likely that particular person is to offend again. That is put together based on an algorithm created by a private company and based on 183 questions. Although race is not an explicit question in the questionnaire, the algorithm tends to favor non-black offenders. As another example, Amazon created a recruiting algorithm where it would look at CVs and output the people most likely to be successful at Amazon. The algorithm turned out to be extremely biased against women, discounting resumes that had the word “women” such as “women in tech advisory board.” It also discounted women’s colleges. This shows how AI can amplify bias.
In this renaissance of AI, is it indeed still garbage in garbage out? Is human bias still reflecting in algorithmic bias, or is it just the accuracy of the algorithm?
From Rana’s perspective, this is a very complicated discussion. There are many multiple facets when you’re looking at bias. AI algorithms affect our lives on a day to day basis and their presence is far more prevalent than people realize. AI based decision making algorithms could determine a simple aspect of approval for insurance coverage or a loan application from a bank. The algorithm is likely making a decision based on your risk profile, and perhaps even race and gender. You are being profiled in terms of what your projected longevity. When you’re building an AI solution, you have an incredible amount of responsibility to ensure that you’re building it bias free. Typically you build models based on specific data sets. You require a tremendous amount of data and subject matter expertise needed to fine-tune the model, and that is where the biases creeps in. For example, when marking video files, a human expert would be involved. If you don’t have a very stringent mechanism to annotate a video or audio file, bias may build up. The cultural aspects linked to the individuals who annotate the files may be reflected in how they see those data points.
Annotating an audio file may be one of the simplest aspects we could talk about. There are vastly more complex aspects we could get into. You thus need to select a group of individuals with more cross-cultural, different distributions of gender, age, geographies. It’s hard to do, and not all systems follow these standards. For an AI algorithm to be effective, it has to be without bias. That is the main incentive to do it the right way for developers and companies as otherwise it wouldn’t work and be ineffective in terms of the problems we are looking to solve. That said, we have an alternate dilemma. When we aim to build a self-learning system, which has capabilities that are as close to a human as possible you’d have this system as perceptible to developing a bias as humans are. You can take an unbiased self-learning system, and it would quickly become biased based on the environment. The dilemma for AI developers is: would you allow that self-learning system to develop bias as a natural human? Or would you influence it to inject capabilities to make sure it doesn’t get biased? Which one is moral? Which one is the right way of doing things?
What about the use of AI in vaccines? Is it compressing certain testing stages, or is it providing better prediction for those testing stages?
Ayla Aynnac works on creating anyone of a heart tissue sample having a piece of genomic and immune system information inside that is utilized as a preclinical. AI allows us to predict the human response before the clinical trial, and it is essential considering the failure rate of drugs (40% of drugs are failing). That is a considerable investment loss, and success rates are in the one digit. In heart failure, not many successes are happening. AI is training based on the human donor heart, and the patient’s created tissue heart, bringing data from the real human to the created tissue, and prediction power is getting higher. Genetic differences will come much quicker into the response as we get access to human data and improve drugs’ development with better matching. AI is critical to decrease drug development costs and match and certify the patient population. The drugs need to correspond to the human. If they have a heart failure condition, cancer patients are at a much higher risk of getting heart failure if it is not looked at properly. How to make sure that the COVID vaccine will match the populations with genetic differences in vaccines? That’s where AI could help solve the problem, and AI has to be trained on humans continuously and evolve to match the human response. And finding the perfect drug requires collaboration. AI will also open the door for drugs for children.
Can you unpack the differences between supervised, unsupervised, and self-supervised learning?
Unsupervised learning is when you show some objects, and you try to organize them by categories. Supervised learning is when you provide directions on the labels. According to Julien’s experience, we generally have the problem in AI that current algorithms aren’t able to learn from little examples. The more examples we have, the better it gets. If we want to train an AI system on images from scratch, we have to provide millions of images, which is not very practical. We have some ways to around it because we can pre-train your algorithms with generic learning to more specific/specialized learning. There still is quite some data that you need. In the last few years, there has been some evolution in self-supervised learning. You give some exercises to the machine where you don’t need so much extra data. For example, in NLP and language analysis, you can take Wikipedia, blank out some words, and ask the machine to guess the words. If it guesses well, it will start to develop a better understanding of the language and our world. For images, you can rotate them randomly and ask the system to figure out the original orientation. Babies do the same. This is great because it allows you to reduce the amount of data you need, to a fraction, around 1%. And maybe we’ll be able to democratize the use of AI with fewer data. AI is moving away from automation and is becoming more about prediction.
Indeed, AI models have evolved from descriptive to predictive and prescriptive. AI is evolving from a passive tool to a more generative, adaptive, contextual, and intuitive tool. AI is moving up the ladder of cognitive functions, which leads us to our next observation.
What is the relationship between consciousness, imagination, and intelligence? Are they intertwined in a way that they are indispensable to one another?
If AI is moving up the ladder of cognitive functions, could AI evolve from its current form to develop a consciousness on its own and imagination, in the same way, humans think? What is the threshold from which consciousness starts to emerge in a system or organism?
In his book, Goder Esher Bach, Douglas Hofstadter Hypotheses that if consciousness can emerge from the former system of firing in neurons then so too computers will attain this similar form of intelligence.
Since long ago, humans have created tools precisely because they are more skilled than humans at performing certain tasks. That is why we build those tools in the first place. Archimedes once said: “give me a lever, and a place to stand, and I will move the earth. Give me a firm spot on which to stand, and I shall move the earth.” The tools we create empower us. What is different from artificial intelligence? In evolutionary terms, computing and artificial intelligence is a relatively new discipline. The first computers emerged in the 40s and 50s, and since then, developments have been exponential. The pace of change is unprecedented. In particular, with the advent of cloud computing, the convergence of AI, Cloud computing, Big Data Analytics, and the Internet of Things is creating a fertile ground for disruptive innovation at an unprecedented pace.
And since humans continue to operate AI, we could argue that artificial intelligence is meant to augment human intelligence rather than replace it. If AI could imagine a complex game like chess or go, and invent a second-order AI that could beat the first order AI at the game created, would AI be at par with human intelligence?
A famous leader once said that imagination rules the world. If imagination rules the world, then will AI ever rule the world?
Although such a dystopian future might be distant, we should be concerned with the potential unethical use of AI, and the risk of “ruling the world” using AI as a proxy. Mass surveillance using advanced AI and facial recognition presents several ethical challenges and raises serious ethical questions. AI will do what we tell it to do, so we should be careful about what we tell it to do.
AI encompasses technology, policy, ethics, economic, social, philosophical considerations. The COVID situation made us realize the criticality of finding the right synergies between technology and appropriate policy responses. We should also develop the correct narrative so people understand the benefits of artificial intelligence for society as a whole. We should implement policy and regulation to raise awareness and unlock the potential of technology in a way that benefits as many people as possible. AI can solve some of the most complex and challenging problems humanity faces and work towards the greater good.
Article authored by:
- Gurvinder Ahluwalia, Founder and Chief Executive Officer, Digital Twin Labs, USA
- Ayla Annac, Chief Executive Officer, InvivoSciences, USA
- Marta F. Belcher, Partner, Ropes & Gray, USA
- Rana Gujral, Chief Executive Officer, Behavioral Signals, USA
- Jean Lehmann, Chief Executive Officer, Cyber Capital HQ, United Kingdom
- Julien Weissenberg, Founder, VisualSense AI, Switzerland